‘What do you mean you haven’t heard of ChatGPT? How can that be?’
This is likely to be the reaction of many first-time users of ChatGPT when they encounter people who have not yet had contact with OpenAI’s technology. The subsequent descriptions of the bot’s abilities then indeed lead to astonished faces almost without exception and hardly anyone remains unaffected by this special moment of ‘AI-enlightenment’.
The astonishment is not without reason, because in fact ChatGPT is able to perform tasks in a level of detail that was reserved for previous language models. Examples of these tasks are:
- Summary of technical texts in the style of a particular author
- Generation of stories and poems
- Creation of program code based on natural language descriptions of a software functionality
- Recipe suggestions based on a list of ingredients
These and many other use cases with ChatGPT playing through is immense fun and and gives you an idea of what’s to come in the future. However, it is precisely this ‘I see’ moment that also leads to misconceptions about the capabilities and use cases of the bot. In this blog, we want to clear up these misconceptions and shed some light on the opportunities and limitations of the bot.
ChatGPT - Deep Dive to Deep Learning
Anyone who has followed the evolution of language models and chatbots in recent years will also be familiar with the case of Google’s LaMDA(Language Model for Dialogue Applications). The chatbot made front-page news in June 2022, when software engineer Blake Lemoine went public with chat histories that allegedly prove the chatbot had gained consciousness.
Even if the majority of experts agree that the bot’s statements are not an expression of consciousness, the model was at least able to pass the Turing test, i.e., it was able to convincingly appear as a human interlocutor to a human being.
One may assume that this persuasive power will only increase with better and better language models. Humans will no longer be able to identify an AI as such in many deployment scenarios (except through very specialized questions aimed at the AI-human distinction). However, this more and more disappearing distinction between humans and machines also holds the greatest pitfalls and dangers in the use of AI: We attribute to AI, because of its human expressiveness AND its technical origin, properties that it does not possess at all, or only partially.
The false underlying assumptions that often resonate with its use are as follows:
In the public testing phase, ChatGPT 3.5 has so far been a static model, meaning that the data (and facts) it has been trained with potentially have an expiration date. The training data of the model in version 3.5 includes data until 2021 and includes software documentation, program code and various sources from the field of “Internet phenomena”. Thus, it is quite possible that ChatGPT may make no or incorrect statements about specific content that was not part of the training.
With the integration of ChatGPT-4 into Microsoft Bing, the situation has changed fundamentally. As an extension of the search engine functionality, the system now connects to current and updated data from the Internet. The model can use it to return and summarize search results and tasks based on up-to-date information. Whether and to what extent this real-time data is used to continuously optimize the model itself cannot be determined at this time. However, it’s safe to assume that user feedback will be an important source of optimization for the bot (as it has been in the past).
The correctness of statements made by the bot can NOT be guaranteed. There are two possible reasons for this:
- Either the bot does not have an appropriate fact base (because the data was not included in the training or in a search result)
- The bot has an appropriate fact base, but reproduces it inadequately or incorrectly
Evidence of this behavior has recently been offered by the chatbot demonstrations from Microsoft (ChatGPT) and Google (LambDA/Bard) themselves:
Although the model has access to the correct facts in the examples, it was not able to provide the correct answers. This behavior is related to the fact that the model does not treat facts like a static database, but treats information as part of its neural network architecture, which brings us to point 3:
The bot’s responses often suggest that it has a deep understanding of the factual relationships in the data. In fact, so-called“reasoning” – the ability to draw deductive and inductive conclusions and make predictions based on a data set – is one of the most amazing features of large language models. Such language models are based on neural methods/deep learning, so they function similarly to a human brain. To a certain extent, they are able to recognize correlations between object properties and make generalizations based on the processed data – and this only on the basis of the presented data and the linguistic concepts contained in them.
Here is an example: When ChatGPT is asked for the definition of Mug, it gives the following answer:
“A mug is a cylindrical-shaped cup with a handle, typically used for drinking hot beverages like coffee, tea, or hot chocolate. Mugs can be made from a variety of materials, including ceramic, glass, plastic, and metal, and can come in a wide range of sizes, shapes, and designs. They often have a capacity of around 8 to 12 fluid ounces (240 to 350 milliliters), but larger and smaller sizes are also available. Mugs can have various features like lids, insulated walls, and decorative patterns or prints.”
From the description, however, it is not possible to tell to what extent the description is the result of a learned abstraction over the concept “cup”, or whether the bot is simply using fixed, existing definitions of a cup in the training data here. Via Google search this specific description could not be found at least. Also, the partial sentence “A mug is a cylindrical-shaped cup with a handle” cannot be found in this way, whereas the sentence “A mug is a cup with a handle” can be found frequently. ChatGPT has the object property “cylindrical shaped”, so possibly as a generalization itself derived from the training data.
In the meantime, a number of research projects have been devoted to the identification of these capabilities. As astonishing as these model capabilities are, it is also clear at the same time: A real understanding in the sense of a human subject is not present here. Only the well-formed presentation of linguistic symbols, together with our associations and projections, creates the illusion of a thinking subject. A large part of this illusion is contributed by ChatGPT’s optimization process, which is based on Reinforcement Learning from Human Feedback (RLHF) based. In fact, to generate the most human and specific statements possible from the bot, the system was continuously evaluated with the help of a very large number of human collaborators.
Responses from the bot were classified into desirable and less desirable responses using a rating system. Based on this feedback, the bot has learned to generate the desired statement form for specific facts. User feedback gained in interactions will continue to be an important source of system optimization.
Conclusion
Many application areas have already emerged in which ChatGPT or ChatGPT-like systems can revolutionize entire fields of work. This includes not least software development. But teaching and the creative sector will also feel the influence of the models: wherever texts are to be summarized or generated without resorting to the same text modules over and over again, language models will sooner or later find their way in. Despite all the possibilities (and dangers), however, we should not forget that the models do not represent a general AI that is capable of independent thought or even consciousness.
ChatGPT aptly summarizes his skills when asked about his deductive ability as follows:
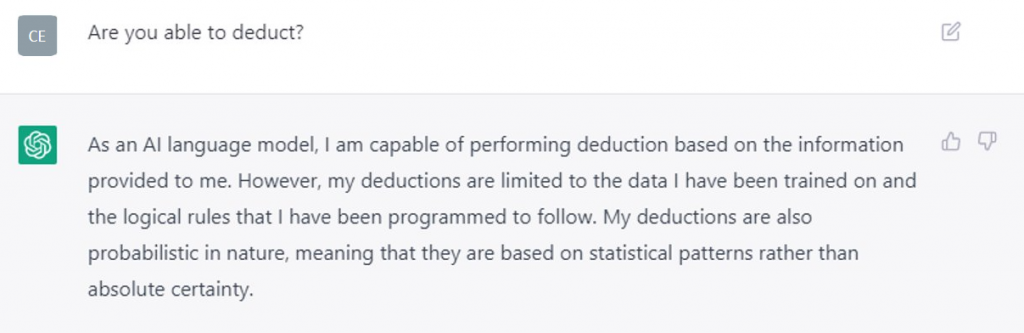
Thus, if the bot were merely fed data from fantasy novels in which laws of nature unknown to us prevail, the bot would learn and reproduce them in the same way as it learns and reproduces the scientific relationships it receives from its non-fictional training data. Despite the truly amazing performance of the bot, there is still a long way to go before the system can evaluate the correctness of its own statements, let alone the factual basis. Here, he is just as reliant on trusting his training texts as a human user of Wikipedia. Or can you always determine beyond doubt whether an author in Wikipedia has made a mistake?